Your single-model AI strategy is costing you millions
In the race to adopt artificial intelligence (AI), telco executives face a critical decision: Should you use a single LLM for everything, or deploy specialized models for different tasks? Some cloud providers push for the simplicity of a unified approach, but here’s what they’re not telling you about the real costs—and limitations—of the one-model-fits-all approach.
The generalist approach—using one model for everything—sounds great on paper. A single, powerful model handling everything from customer chats to network analysis promises consistency, simpler deployment, and streamlined vendor management. But here’s the catch: you end up paying premium prices for every task, even basic ones. It’s like using a supercomputer to send emails.
The specialist approach flips this thinking on its head. Instead of forcing every task through the same expensive model, you deploy different models optimized for specific needs. For example, choose a fast, lightweight, cheaper model for millions of customer service interactions where speed is critical, and a sophisticated model for network optimization where accuracy could mean the difference between service disruption and smooth operations. Lower costs, better performance—but yes, with more complexity to manage.
Why do you need a specialist approach?
As you dig deeper into using AI across your operations, your approach becomes increasingly critical. Let’s look at three real-world scenarios that expose why model choice matters. In the following examples I assume a premium model cost of $0.01 per 1K token vs. a specialized model cost of $0.0005 per 1K tokens—a 20x price difference. For a more in depth analysis of AI model pricing, see the website artificialanalysis.ai.
1. Network operations: when volume drives cost
![]() |
Your network generates an avalanche of data—system logs, performance metrics, security alerts, resource usage stats. With one terabyte (TB) of network data daily, running all this through a premium LLM would bankrupt your digital transformation budget by lunch. |
One TB of network data daily generates 50-million log entries with 100 tokens each—for a whopping five billion tokens every day.
Cost Comparison:
- Premium model cost: Daily cost: $50,000 ($0.01 × 5B/1000) ➜ Monthly cost: $1.5M ($50,000 × 30)
- Specialized model cost: Daily cost: $2,500 ($0.0005 × 5B/1000) ➜ Monthly cost: $75,000 ($2,500 × 30)
Using an all-premium model approach would cost $1.5M per month, but smart routing with 95% specialized models cuts that to $150K, saving you $1.35M monthly.
2. Customer service chatbots: speed kills (your budget)
![]() |
When you’re handling millions of customer queries daily, every millisecond of response time affects customer satisfaction—and every penny spent impacts your bottom line. Using a premium model like GPT-4 for basic customer queries is like hiring a PhD to answer phones. |
A mid-sized telco handles about one-million customer queries monthly, with each query using around 200 tokens.
Cost Comparison:
- Premium model cost: $2,000/month ($0.01 × 200 × 1M/1000)
- Specialized model cost: $100/month ($0.0005 × 200 × 1M/1000)
Using a cost-optimized model, you’d save $22,800 per month with this approach.
3. Legal and compliance: accuracy above all
![]() |
For reviewing spectrum licensing or ensuring regulatory compliance, you absolutely want to pay for premium model accuracy. A mistake here costs far more than any AI savings. But here’s the key—you need this premium processing for only a fraction of your total AI workload. |
Out of one million documents processed monthly, only 50,000 (5%) are critical regulatory documents that require premium accuracy. Assuming an average of 500 tokens per document:
Cost Comparison:
- Premium model: ➜ Per document cost: $0.005 (500 tokens × $0.01/1K) ➜ All premium approach: $5,000/month ($0.005 × 1M docs)
- Selective approach: ➜ Critical docs (premium): $250/month (50K docs × $0.005) ➜ Regular docs (specialized): $125/month (950K docs × $0.00025) ➜ Total cost: $375/month
Using a cost-optimized model, you’d save $4,625 per month.
Add it all up
A mid-sized telco could save millions annually by taking a specialist approach to AI model selection. With numbers like these, you might wonder why every major cloud provider isn’t embracing this strategy. But just follow the money: Microsoft’s $13 billion bet on OpenAI essentially locks its customers into GPT-4 and its derivatives. Google’s doing the same with its Gemini family. When they ask you to use the same model for everything, they’re asking you to consistently overpay for basic operations while potentially compromising performance where it actually matters.
Enter AWS’s game-changing approach
While others push single-model solutions, AWS is building an ecosystem that makes specialist AI practical at scale. At re:Invent 2024, AWS doubled down on this strategy with its expanded model marketplace and intelligent routing capabilities. The result? A platform that automatically matches each task to the most cost-effective model, cutting costs by up to 30% while maintaining performance. What makes this approach powerful isn’t just the 100+ models available—it’s how AWS has solved the complexity challenge. Its platform handles model selection and optimization automatically, giving you the cost benefits of specialized models without the management overhead. For telcos, this means you can focus on outcomes rather than juggling AI models.
The real cost of choice
When Microsoft and Google pitch their limited model offerings, they’re not just selling you AI—they’re selling you constraints. Sure, having two or three models might seem simpler than navigating AWS’s extensive catalog. But in an industry where margins matter and performance is non-negotiable, artificial simplicity comes at a very real cost: $16.5M annually for a mid-sized telco.
In the real world of telco operations, you don’t use the same tool for everything—and you shouldn’t use the same AI model for everything, either. The future belongs to telcos who understand that AI strategy isn’t about picking the best model; it’s about picking the right models for each job. The question isn’t whether to take a specialist approach anymore. The question is: how much money are you willing to waste before you make the switch?
Recent Posts
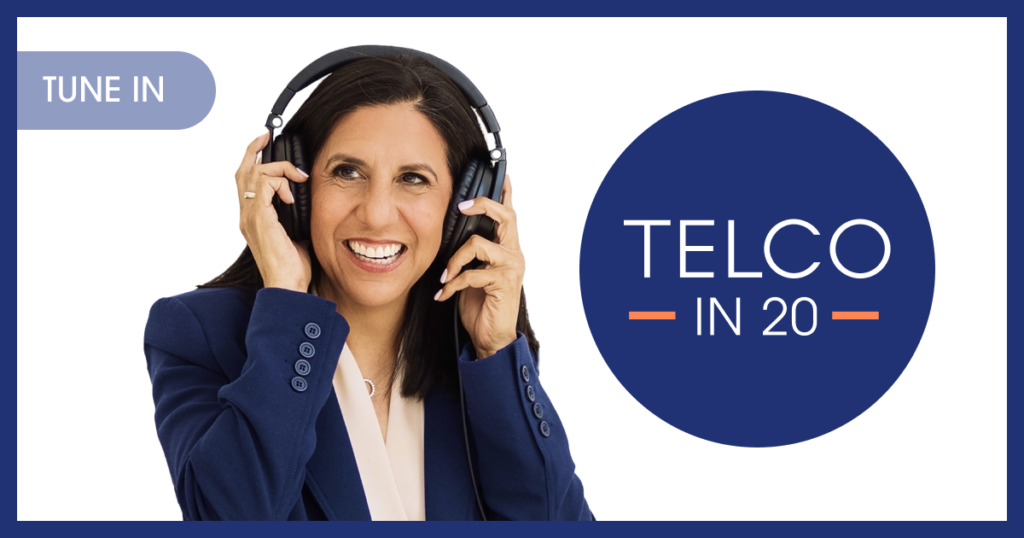

Get Started
Contact Totogi today to start your cloud and AI journey and achieve up to 80% lower TCO and 20% higher ARPU.
Explore
To make smart decisions about AI tech, telcos need to clear up confusion around overused buzzwords.
Engage
Set up a meeting with the Totogi team to learn how to implement a specialist approach with BSS agents built on any of your BSS applications.
Try
Sign up for a pilot of Totogi’s BSS Magic to find out how you can transform spoken ideas into a robust, custom BSS—no engineering expertise required!