How to transform your customer support with AI
Pretty much everyone agrees that customer support is the first place to look when you’re ready to add generative AI (GenAI) to your organization. Because this function has high volumes of repetitive tasks and clear performance metrics, it’s an easy place to start. Implementing it is usually straightforward, and it delivers immediate, measurable results. Plus, barriers to putting GenAI to work in customer support are relatively low compared to other areas, which means companies can increase efficiency and customer satisfaction with minimal change to existing processes. All of this makes customer support an ideal launching pad for broader AI integration.
With the GenAI explosion of last year, I wanted to find out if we could boost performance across Skyvera and Totogi by using GenAI in our own customer support function. So, a little over a year ago, we set out to answer the question, “how many of our support tickets could be completely handled by GenAI?” At the time, our workers were managing 100% of approximately 1,700 new tickets per week, and we wondered if we could get GenAI to handle half (or more) of them.
Today, almost a year later, AI has revolutionized Totogi’s customer support. We not only hit our goal of 50%—we exceeded it, and now more than 56% of tickets are resolved with no human intervention required (and that percentage is still increasing!). I know that at 1,700 tickets per week we are small potatoes compared to telcos’ customer service function. But our improvement trajectory cannot be ignored, especially if you think about the potential savings for your organization. So, here’s the (kind of ugly) story of how we did it.
Getting started
To be super honest, starting was the hardest part. Everyone was fighting about how to approach it, where to begin, who to involve, which tools to use, what products to buy. We had discussions about:
- Whether we should we build our own large language model (LLM)
- How to protect our proprietary data
- The cost of API fees for calling the LLM
- What we would do if people didn’t want to use it
- What to do if it made things worse instead of better
- How to shut down our small thinking so we could get to that big, hairy, audacious goal (BHAG) of 2x productivity
… and on and on. After what felt like 100 hours of discussion among the leadership team, we finally put a stake in the ground and said we would just start.
We contacted our vendor, Zendesk, and it was behind, too. We looked around at competitors, but pretty much everybody was just adding chatbots, and they all kind of sucked. So, we built some things ourselves, knowing that when/if anybody came up with something better, we could always switch.
At first, we just set everybody loose. It was total chaos. All the reps used their own prompts in ChatGPT, Claude, POE, Bard—whatever anyone wanted to use—and it was kind of a disaster. And then a few people started getting results. We had everyone share what worked, and that led to more gains, as well as a sense of camaraderie, with people working iteratively to share and perfect the prompts. Then, we standardized them.
We consolidated the prompts into a system and started training it on our knowledge bases and closed tickets. At first, we had to have humans watching over it before we sent responses to customers. But, in a short period of time, it got better. We kept getting more and more ideas on how to make it more effective, and we created a GPT that would “grade” the answers so we could remove the human quality bar.
Measure your results
In implementing AI, I believe it’s super important to measure the results you’re getting each week. It keeps the team motivated and actually shows everyone that this new change is working. Deciding which metric we would use took some discussion. Would it be the speed of closing tickets? Improvement in our first-response resolution? Reduced headcount? We finally settled on the number of tickets resolved 100% by AI, correctly, with no human intervention. To us, success means no human ever saw the ticket, and the customer gave it a favorable rating. So, that’s the measure we use today.
Take a look at this chart: this was our actual weekly journey. At first, the numbers were anemic: for weeks, no results at all (we started in week 20 of 2023). Then we got 5% improvement, 8%. Sometimes, we’d mess something up and go backward. It was slow and demoralizing for months. But then we had a breakthrough: 12% to 24% in two weeks’ time. And then 31% to 46% a few weeks later.
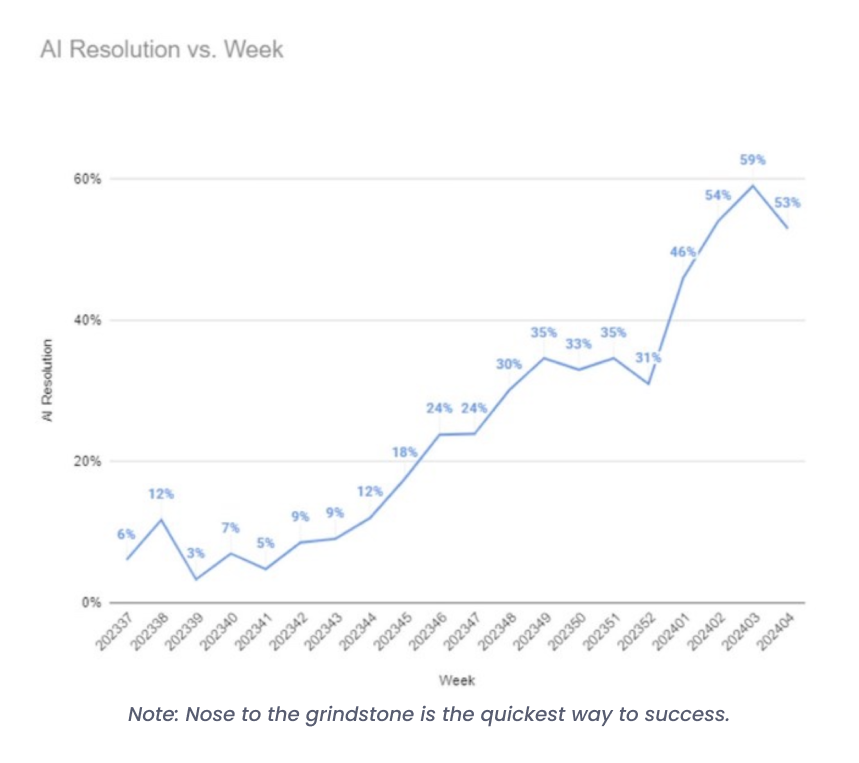
Today, 56% of our tickets are being solved by AI. It took nine months. That’s not a long time in the grand scheme of things, but it felt like forever. And we never would have gotten here if we hadn’t done everything else before—even the chaos, no progress, and backsliding. It would have been easy to give up when we were stuck. But we kept at it. That’s the secret. Like Dory sings in Finding Nemo: “Just keep swimming, swimming, swimming.” 🐠
Motivate your people
A final reminder—don’t forget to involve the people impacted by this kind of change. This will inevitably be a large disruption to their daily workflow, and you’re going to have detractors, naysayers, and Debbie Downers. At first, they’ll say they’re not more productive with AI, that using it slows them down. They’re going to be scared that layoffs may follow if they help implement AI. The thing is: you need to remind them that being good at AI is the best way to not be fired by AI. Learning how to become a kick-ass, AI-assisted human is the reality of everyone’s future. Don’t fight it; jump on board and learn how to be more productive with it.
Be sure to find the champions of this change and reward them and promote their work. Give them a new title to make them the head of AI for their level or group. Internal incentives can unlock new behavior. We gave people small bonuses when they had breakthroughs, and asked the individual contributors who were leading by example to take on special AI projects. This showed others how alignment to the mission was being rewarded. After the organization saw the results and rewards, more and more people jumped on board. Now, our people love to work with AI and don’t want to go back to the “old way” of working. They’re now superpowered, AI-assisted humans. Yay!
What about the quality?
A question we get a lot of the time is, “That’s great, but how’s the QUALITY of the AI output?” It was our experience that AI answering tickets outperformed our below-average workers. For the people who are average or above average, it improves their work and productivity, and for the top 10% of workers, we still have not been able to create AI that exceeds their performance … yet (we are still trying, though!). But the thing is, AI is getting better and faster every day, and we believe it’s only a matter of time before it does a better job than everyone on the team.
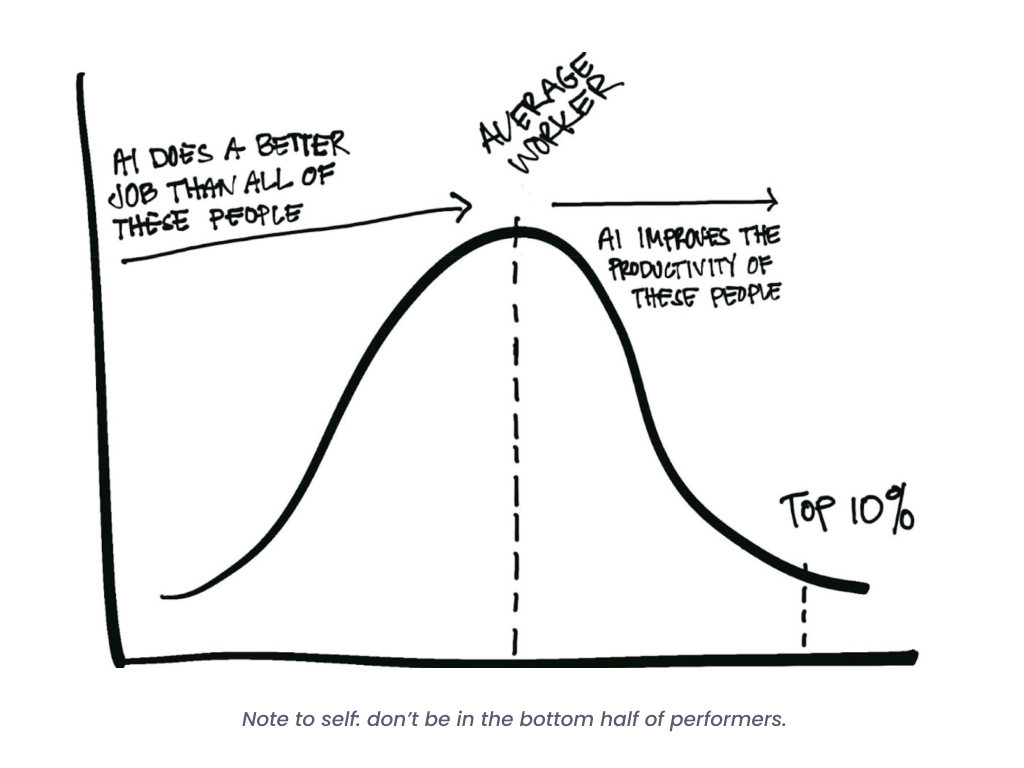
Start eating the elephant
If you’re looking for a silver bullet, you won’t find it here. You’re not going to install AI on day one and watch productivity shoot sky-high by day two. But like my mom always says, “How do you eat an elephant? One bite at a time.”
Start with finding the right tools. If you contact your vendor and the tools aren’t there or are subpar, don’t let that stop you. Look at competitors. Build a basic tool internally. Don’t wait. So what if it isn’t perfect? You’ll be learning what you need and what works for your org, and you’ll be socializing the change with the workers. If the right tool comes out later, you can always switch. In the meantime, Software-as-a-Service (SaaS) products are your friend. Use those free trials and monthly subscriptions to avoid committing to something that doesn’t work for you. You may have to kiss a lot of frogs before you find your prince.
Next, set a goal and start tracking those metrics. Our goal was 2x productivity. Maybe for you, it’s 30% improvement, or 60%. Whatever your target, the numbers will be small at first. They’ll go back and forth. Don’t sweat it. This is what it’s going to be like with all things AI—churn prediction, network anomaly detection, etc. You’re going to think you’re ready, and then realize that your data isn’t ready, clean, or complete. The tools are new; they have limitations. The technology is in massive flux, changing every day. Your graph won’t be going up and to the right all the time, but the trend line will be generally positive. It takes consistent work, and then you get a breakthrough. Voila!
So, get started today! I know you’ll find that using AI for customer support delivers happier customers and new, bigger opportunities for your team and your business. It’s a win-win-win! So, go get it.
Recent Posts
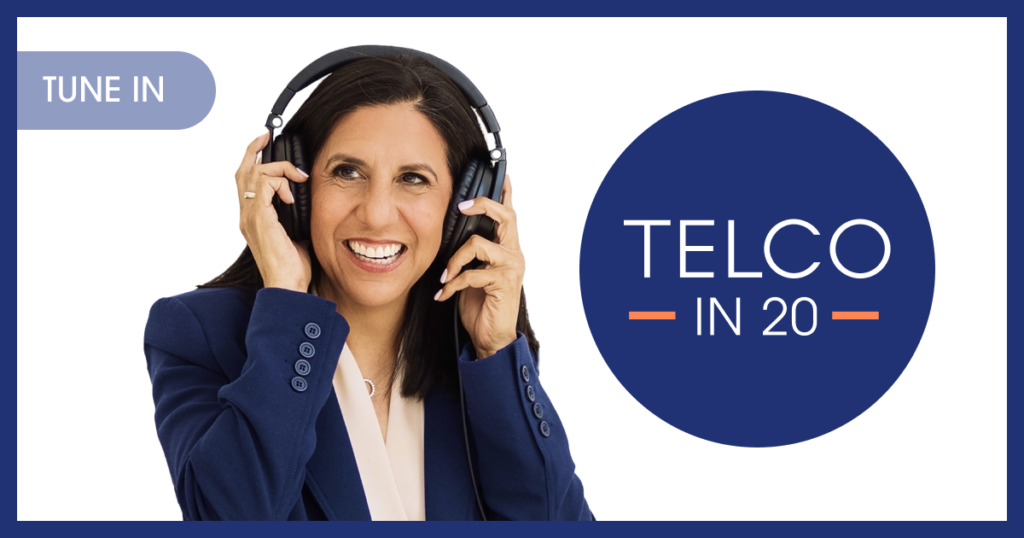

Listen
BT Group started its public cloud journey in 2019, putting it in a strong position to tap the strategic capabilities of AI. Chief Architect, Digital, Josie Smith talks about BT Group’s mission to simplify thousands of apps, unleash AI, and reskill its workforce for digital transformation.
Watch
AIOps is an established concept in the IT industry but a relatively new one to telcos. Deployed correctly, ‘AI operations’ can facilitate end-to-end network automation and zero-touch operations, accelerating decision-making with a more agile and reactive intent-based approach.
Read
AI will be everywhere at MWC 2024. There are companies doing AI right and others that are faking it. Check out the top ways to identify #fakeAI.