Getting AI right is hard 🚀
The honeymoon phase with artificial intelligence (AI) is over. As organizations grapple with the complexities of integrating AI, many are finding it’s not the magic bullet they hoped for. But don’t give up! Instead, take a page from one of the most innovative thinkers of our time: Elon Musk.
When Musk set out to revolutionize space travel, he faced a problem that seemed insurmountable: the astronomical cost of rockets. Instead of accepting the status quo, Musk applied first-principles thinking, breaking down the problem to its most basic elements. He questioned every assumption, analyzed the raw materials, and rebuilt the entire process from scratch. The result? SpaceX managed to reduce launch costs by a factor of ten, making space travel more accessible than ever before.
This same approach—first-principles thinking—is exactly what you need to tackle the challenge of integrating AI into your telco. Just as Musk reimagined rocket construction, we need to reimagine every business process with AI in mind. It’s not about slapping AI onto existing systems (chatbots and copilots aren’t the killer application of AI, for example); it’s about fundamentally rethinking how we operate every process and system within a telco. And that’s hard to do.
The challenge of implementing AI
Recent earnings reports and industry feedback reveal a sobering truth: enterprise AI adoption is progressing more slowly than anticipated. Take, for example, Microsoft’s reset of expectations on AI revenue growth in its latest earnings report. While AI has made solving many hard problems easier, implementing AI itself in a way that provides real business value is kinda hard.
Whenever my team at Totogi is facing a hard challenge like this, we apply first-principles thinking. Like Elon Musk, we methodically break down a problem to its most basic parts, and then build a new solution from the ground up (like say, Totogi Charging-as-a-Service, which redesigned a real-time charging Online Charging System 100% for the public cloud). The idea is to simplify as much as possible, which frees you to focus on the most important elements. It encourages you to question assumptions, start from scratch, and think creatively to find the best possible answer. Just like Elon.
First-principles thinking is most useful when you’re solving a new problem, trying to find a new way to solve an old problem, dealing with complexity, adapting to a new environment, or wanting to be the first to jump on an emerging opportunity… just like AI.
Common errors in first-principles thinking
While first-principles thinking is a powerful tool for implementing AI, it’s easy to fall into some common traps. Here are two of the most common errors people make when applying this approach:
- They fail to really break down the problem, and
- They anchor to existing solutions.
Failing to break down the problem is often the most consequential error. People frequently stop at surface-level assumptions instead of digging deeper to the most fundamental truths.
For example, many organizations get stuck on the high price tag associated with AI tech, viewing it as fixed and unchangeable (as discussed in my recent podcast with Appledore Research’s John Abraham, where he mentions that telco execs are concerned about the high cost of AI). However, with true first-principles thinking, you would not worry about costs; instead, you would break down AI costs to their fundamental components: computing power, data storage, algorithm efficiency, and so on.
By taking this approach you might realize that while current costs are high, the trajectory of these components suggests dramatically lower costs in the near future (see tweet below showing that in just 18 months, AI costs have plummeted 240X for just GPT-4 alone). While the AI Wars are being duked out, you don’t have to worry about the cost problem. Focus on the fundamental problem you are trying to apply AI to, use AI “wastefully,” and cost optimize later when prices have settled at the bottom.
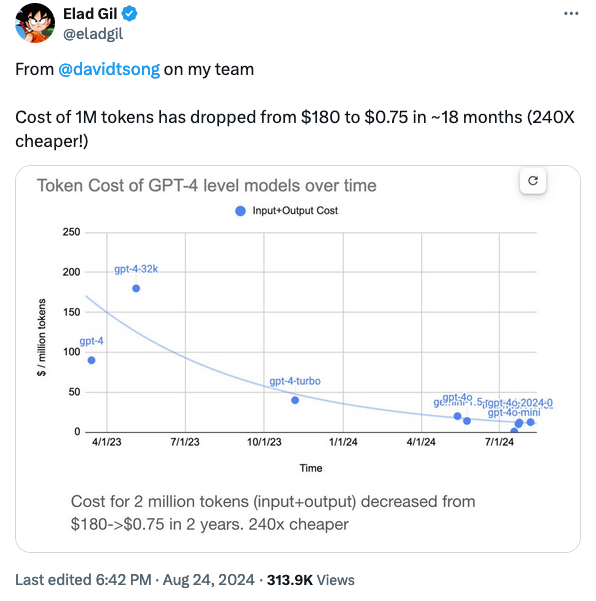
The other problem I see: anchoring to existing solutions. Even when attempting to start from first principles, many struggle to fully detach from old approaches, current solutions, or conventional wisdom. This can severely limit creative problem-solving and prevent truly innovative ideas from emerging.
When applying first-principles thinking to AI integration, teams often subconsciously anchor to their existing in-house solutions or methodologies. They might break down the problem, but their analysis is colored by preconceptions about what solutions should look like—specifically, that they should be developed with existing tools, or done internally by their own teams. Your old vendors may not have all the right answers, especially in the age of AI. This bias can lead to dismissing external, new AI solutions that could be more effective, efficient, economical—and frankly, just BETTER. True first-principles thinking requires setting aside these biases. Instead of asking, “How can we build this ourselves?” or “What does Amdocs have?”, the question should be “What is the most effective way to solve this problem, regardless of the solution’s origin?” This might lead to discovering that an external AI solution, or a hybrid approach combining internal and external elements, is actually the best way forward.
Betting on the innovation curve
Finally, when building with AI, don’t bet on where the tech is right now; bet on the trajectory, where it’ll be a few years from now. I was in an executive’s office one day and they drew this picture on a whiteboard about how they thought about using the public cloud vendors vs. building their own private cloud:
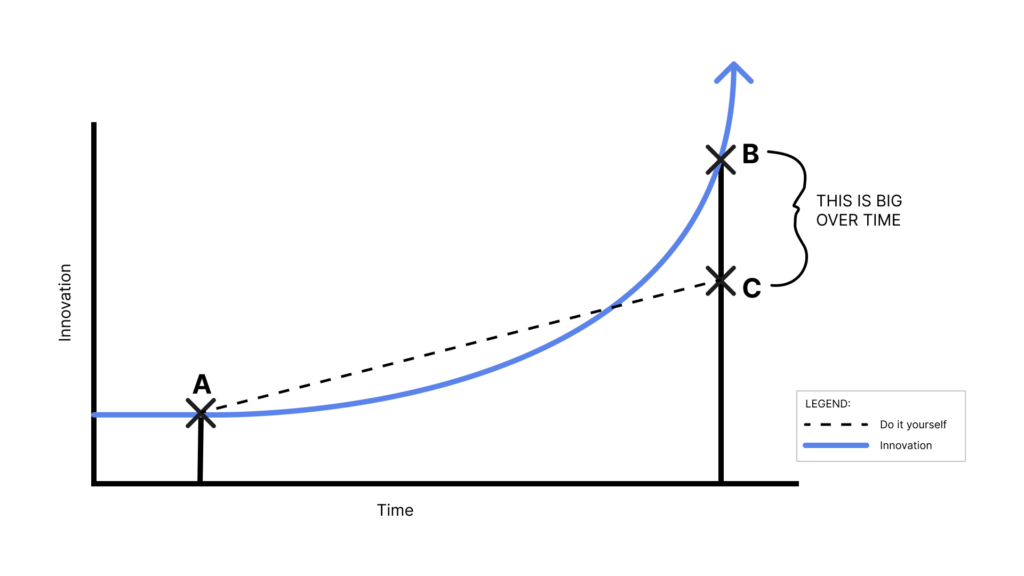
Even if your team can build something better in the short term (A), the long term is what you’re betting on. As the cloud vendors, or AI vendors, keep innovating, investing, and improving, you’re riding THEIR coattails up the curve (B). Why waste time and energy building it yourself, only to end up in a worse position over time (C)?
Even though the example above was for the public cloud, the same is true for AI.
In the age of AI, it’s easy to find a startup that has a swappable component that you can add to your project, see if it works, and keep the project moving along. Plus, you have other important problems to solve, like fine tuning your AI model, integrating it into your processes and systems, and getting your people to use it. Your list is long! Use new vendors’ tech, put it into your solution, and see if it starts getting used. If it doesn’t, then rip it out and throw it away. If it starts to take off, then swap it out for something cheaper or written by your team. You can always change it later.
This is your SpaceX moment in telco
Elon Musk revolutionized space travel through first-principles thinking. Now, it’s your turn to have your own SpaceX moment in the world of telco.
Just as Musk didn’t accept the status quo of expensive rockets, don’t accept the status quo in telco. Break down the problems, question every assumption, and rebuild your processes from the ground up with AI at their core. By applying first-principles thinking, avoiding common pitfalls like cost fixation, NIH syndrome, and betting on the innovation curve, you can lead your organization to new heights. The AI revolution in telco is here, and it’s time to launch your own moonshot!
Who knows? A few years from now, your telco might be the example others use when talking about AI done right. Need help getting started with first-principles thinking for your telco or MVNO? Give me a call, I’d love to help you out.
Recent Posts
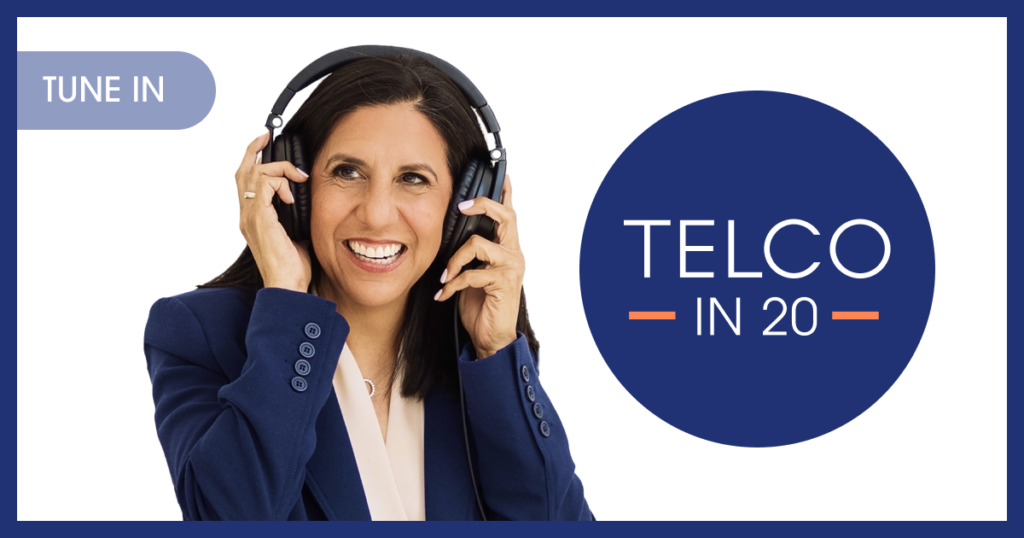

Listen
Canadian telco TELUS is using AI to drive efficiency and innovation across its business. Chief Insights Officer Jaime Tatis shares key learnings and success stories from the company’s transformation journey.
Watch
DR explains how network operators can use AI to harmonise data structures, provide a common language across their often sprawling back-office IT estate, and enable “zero UI” (user interface) interactions.
Read
Think AI is too expensive for your org? Think again. When implemented strategically, AI can pay for itself.