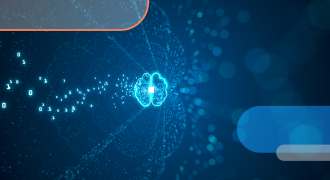
Blog
The BIG problem with generative AI
Generative artificial intelligence (GenAI) is game changing for the telco industry. But can telcos fire it up “as-is”?
If you haven’t yet realized that artificial intelligence (AI) is the future of pretty much everything, you’ve been living under a rock. As a telco executive, you know the competitive advantage AI can bring to your business, from improved network performance to enhanced customer experiences. But creating a plan to deliver AI to your organization is probably keeping you up at night. Hopefully, you’ve already dismissed the idea of building a multi-thousand Nvidia GPU cluster to create your own Large Language Model (LLM), and instead have headed for the obvious choice: using the public cloud.
AI in the cloud, or “Cloud AI,” is ready to make your networks smarter, your processes more efficient, and your customer experiences personalized—at a price you can afford. AI workloads, especially the training and deployment of large-scale models, require immense computational power and specialized hardware. Of course, the scalability of the public cloud is perfect for this. But in this blog, I want to talk to you about the custom silicon chips that are the real secret sauce of Cloud AI. They offer unmatched speed, cost, and innovation benefits to telecom operators that can’t be replicated on-prem.
To start, AI needs speed. Telcos need to process huge amounts of data in real time, whether it’s voice calls, video streams, or network data. You need to train complex models that can learn from data and make predictions. You need to do all this without delays or errors.
The chips of the public cloud are designed to accelerate AI inference and training by orders of magnitude compared to traditional CPUs and GPUs. They can handle billions of operations per second with high accuracy and low latency. Up until now, Nvidia has been king of the hill when it comes to AI-optimized chips, but a battle is heating up among the major tech players. AWS, Google, and others are the new kids in town, and they’re bringing some serious muscle.
For example, as part of a huge release of AI tools, AWS recently announced the general availability of two custom chips that improve performance and reduce costs for high-performance, low-cost inference tasks, helping to put Cloud AI within reach of more companies. Amazon Elastic Cloud Compute (EC2) Trn1n instances powered by AWS Trainium are specially designed for AI training tasks. EC2 Inf2 instances powered by AWS Inferentia2 are designed to speed up AI runtimes and offer 20% higher performance compared to other EC2 instances. Google has also been busy, revealing an AI supercomputer called TPU v4, and says it’s 1.2x-1.7x faster than Nvidia and uses 1.3x-1.9x less power than the Nvidia A100. And rumors are flying about Microsoft teaming with legacy chipmaker AMD as both partners aim to develop their own AI chips, codenamed “Athena,” in an effort to reduce costs and reliance on Nvidia.
Whichever cloud you pick, you should be using these specialized AI chips to make your AI applications fly.
It’s no surprise that AI workloads cost a ton of money. This is due to the substantial computational resources, energy consumption, and specialized hardware required for processing complex algorithms and large amounts of data. Additionally, large data set storage and infrastructure expenses contribute to the overall cost.
Using public cloud custom AI chips for your AI workloads can save money by accelerating AI processing with greater efficiency and lower energy consumption. These chips optimize resource utilization, scale according to workload needs, and reduce the infrastructure and maintenance expenses associated with on-prem setups. This allows organizations to harness advanced AI capabilities while minimizing costs.
For example, AWS Inferentia chips can deliver up to 45% lower cost per inference than GPU-based instances, and Google claims TPU chips can deliver up to 80% lower cost per training than GPU-based instances. Azure is still playing catch-up in this area—currently, its NPU chips deliver up to 50% lower cost per inference over CPU-based instances. With ChatGPT costing Microsoft $0.36 per query, this is going to be a big focus for it to aggressively reduce costs as it steals search market share from Google.
The good news is that all of us cloud lovers will be the winners, allowing regular people like you and me to save big money and optimize our budgets.
Finally, AI needs rapid innovation. You need to explore new possibilities and solutions for your business problems and opportunities. You need to experiment with different models and algorithms that can fit your data and goals. You need to do all of this without spending too much time or effort and, thankfully, the public cloud delivers on this axis as well.
The public cloud chips integrate with various tools and platforms that the public cloud providers offer to help you build and manage your AI projects. These tools and platforms are easy to use, flexible, and powerful.
For example, AWS offers Amazon SageMaker, a fully managed service that enables you to build, train, and deploy machine learning models using AWS Inferentia. You can use SageMaker to create models from scratch or use pre-built ones from AWS Marketplace. You can also use SageMaker to monitor and optimize your models with features like AutoML, Debugger, and Experiments.
Azure offers Azure Machine Learning, which allows you to create, deploy, and manage machine learning models. You can use it to access data from various sources, apply transformations and algorithms, and publish your models as web services or APIs.
Google Cloud offers Vertex AI, a unified platform that provides end-to-end services for building and managing AI projects using Google TPUs. You can use AI Platform to access data from Google Cloud Storage or BigQuery, train models using TensorFlow or PyTorch, and deploy models on Google Kubernetes Engine or Cloud Functions.
By using these tools and platforms, you can accelerate your AI development cycle and stay ahead of your competition.
The future of our industry is more than just 6G. It’s about optimized networks, real-time fraud detection, more efficient processes, and superior customer experiences—and ultimately telcos owning the consumer relationship. With your petabytes of data, the power of the public cloud, and AI specialized chips, it could be YOU pioneering a new way forward. It’s time to go all-in on Cloud AI. It’s not just a nice-to-have. It’s a game changer. The future is calling you, telco. Will you answer?
Recent Posts
"*" indicates required fields